This will hide itself!
And Which AI Is Best For You.
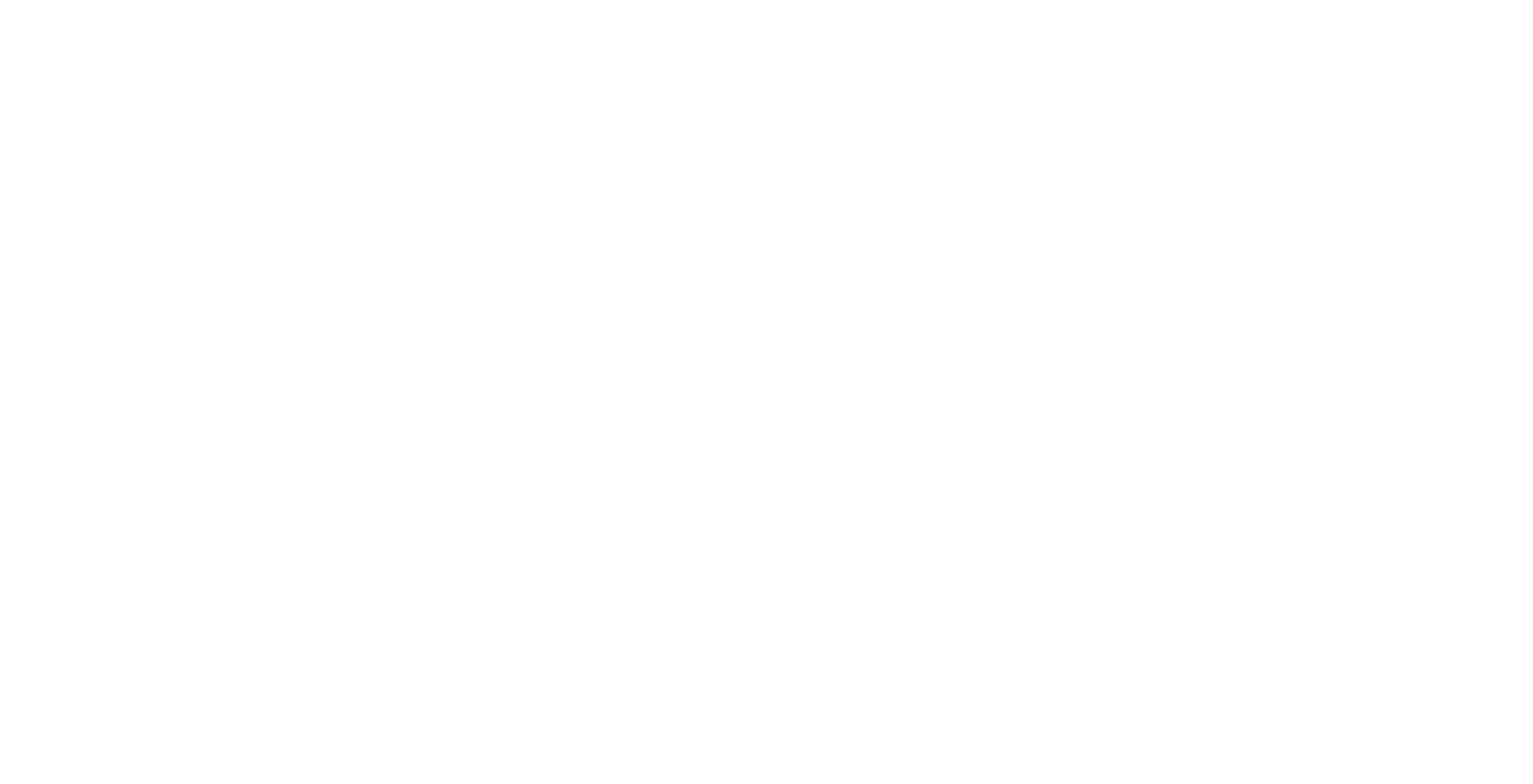
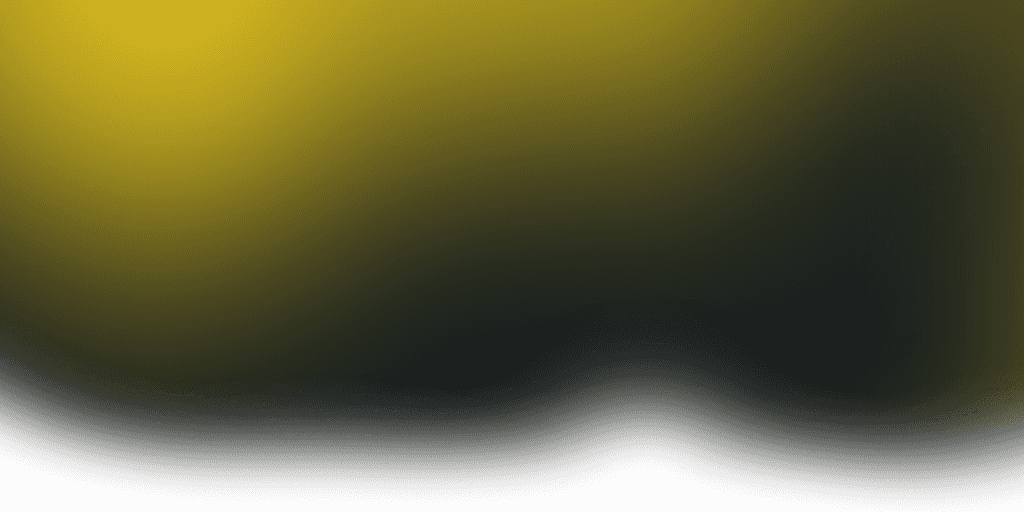
Artificial intelligence has grown in prominence and overtaken the media with new innovations and creative capabilities. Many of us are familiar with AI, which has gained traction for being able to write a song or paint a picture, but what different models are there, and what exactly are they capable of? There are two fundamental models: generative or large language models (LLMs) and focused models, each with its own distinct approach and set of capabilities.
Enter the generative AI, the jack of all trades. These algorithms are designed to tackle a wide array of tasks and are capable of doing anything from writing essays, editing speech, and conjuring images by absorbing patterns and structures from immense amounts of data. Picture them as the polymaths of the AI realm—versed in countless areas but mastering none to its full potential. While generative AI boasts versatility, it's not without its limitations. They require energy and struggle with accuracy in specific tasks. However, with the capabilities to intake text prompts and data and create outputs that mimic manmade speech and creation, generative AI has become increasingly popular, and the model most people are familiar with as modern artificial intelligence.
These models intake immense amounts of data and require considerable training time, which makes them impressive in the realm of possibilities. However, their outputs can sometimes miss the mark in accuracy and focus due to their all-encompassing nature. When it comes to creative possibilities in machine learning, they're the undisputed champions, learning and evolving from data remarkably. Its groundbreaking innovation is no small feat, and its capabilities are beyond anything many of us could comprehend even just a few years ago. With such fantastic discoveries—unfortunately—there are drawbacks as well. When processing items, generative AI requires much more time and energy than focused models, being up to 400 times slower when completing tasks of comprehending data due to the fact its size is thousands of times larger. This taxation translates to large amounts of energy requirements. The data sourced has unclear origins with questionable privacy and copyright implications, and although incredibly diverse, it is not always of high quality. With these limits, accuracy becomes a concern. Training a model in its initial stages requires processing capabilities to comprehend immense amounts of information available, and although this takes a lot of energy and can be very expensive and time-consuming, it is the process of using the model, known as “inference,” that keeps accumulating costs over time.
As dominant companies in the AI market become increasingly profitable, they have also become more secretive in how their models work, where the data comes from, and the energy that they require. Therefore, it is difficult to know exactly how much energy these companies, such as OpenAI, are truly using. A study done by Verge notes that image generation models “used on average 2.907 kWh per 1,000 inferences [...] and the average smartphone uses 0.012 kWh to charge — so generating one image using AI can use almost as much energy as charging your smartphone” (Luccioni, as cited in Vincent 2024). As this continues, there is an environmental threat tied to the widespread use of artificial intelligence, with the requirements for sourcing the resources needed using as much energy output as entire countries. By 2027, the AI sector could require up to 134 terawatt hours each year, roughly the same amount of output as the entire country of Sweden, which requires 137 (deVries, as cited in Vincent 2024). For building quick prototypes and beginning to understand the abilities of artificial intelligence, generative AI is a great step towards the future and a fantastic tool for many, but for long-term applications that require speed and accuracy in the technology available, focused models are a more suitable option.
The focused AI models are the refined specialists of the AI world. These models lack variety in capability in favor of pinpoint precision and efficiency. They are trained on vast datasets with a specific purpose, making them the superior model for tasks requiring accuracy and speed. They're the scalpel to generative AI's Swiss army knife—particularly adept at tasks like sentiment analysis, anomaly detection, and recommendation systems, where expertise and context are paramount. For instance, our model, Asent, is an example of focused AI in the realm of business analytics to better understand customer insights. While generative models may fumble with specific applications, Asent can use the skills designed for such a particular task to provide better speed and accuracy. Its focused approach allows it to navigate the intricacies of sentiment nuances, delivering actionable insights to businesses with unparalleled reliability for such a task. It uses machine learning to continuously improve and understand careful nuance, but it cannot resemble the capabilities of generative AI’s understanding of prompts to create images or text with the goal of resembling human creation.
In the realm of all AI, both generative and focused models have unique advantages and trade-offs. Generative models dazzle with their adaptability across diverse tasks that can seem like magic, while focused models exemplify precision within specific domains. The choice between these models hinges on the task at hand, the resources available, and the desired outcomes. By understanding the strengths and limitations of each approach, businesses can harness the full potential of AI to navigate their individual challenges.